Unlocking the Power of Image Annotation for Machine Learning
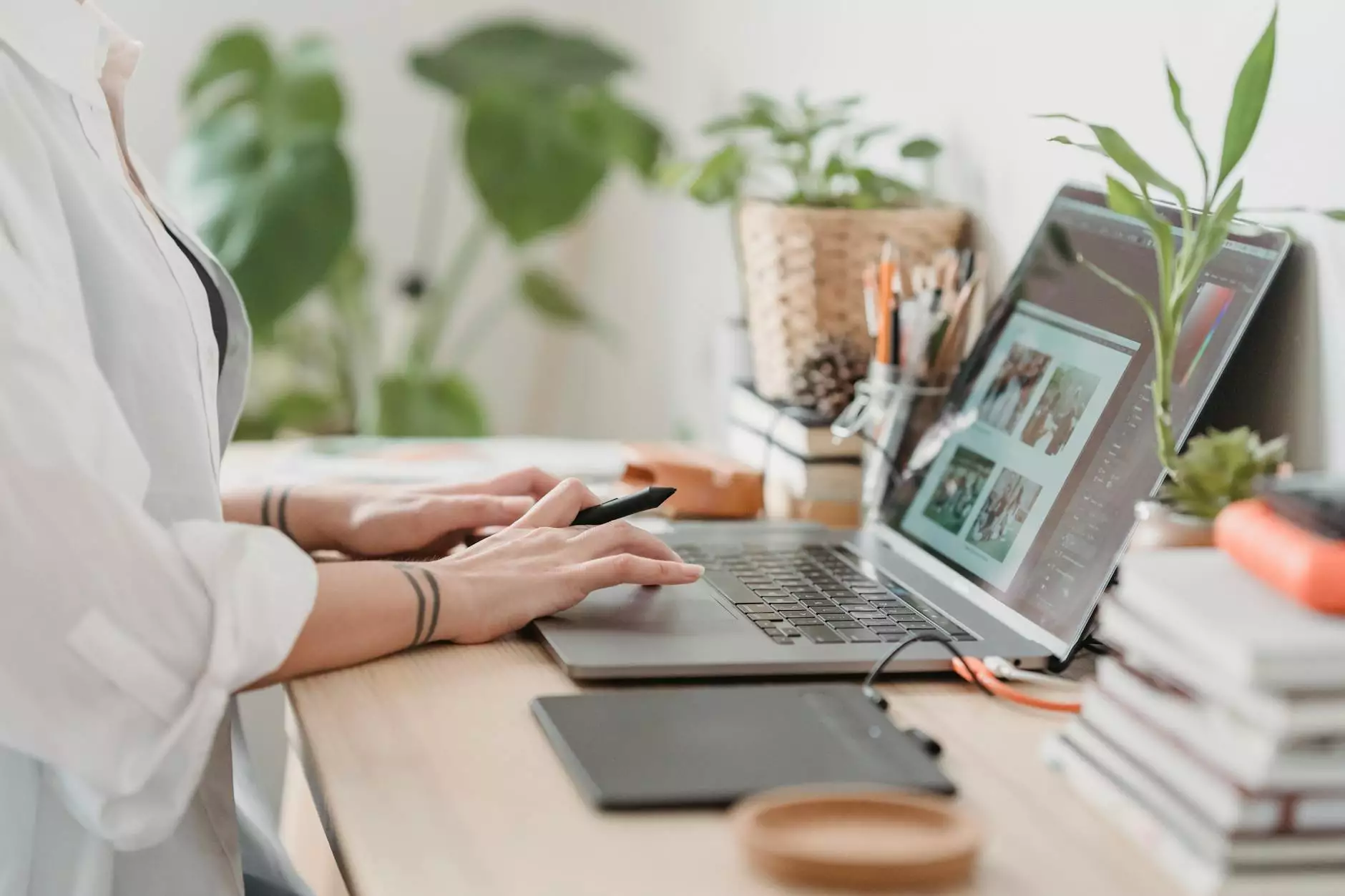
Image annotation for machine learning is a critical aspect of training artificial intelligence models, particularly in the fields of computer vision and natural language processing. Through effective image annotation, businesses can harness vast amounts of visual data, converting it into actionable insights that drive innovation and efficiency across various industries. In this comprehensive guide, we’ll explore the essential steps involved in image annotation, the various types of annotation, and how using a robust data annotation platform like keylabs.ai can give your projects the competitive edge they need.
What is Image Annotation?
At its core, image annotation is the process of labeling images with relevant data that can be understood by machine learning algorithms. This labeling provides the context that machines need to recognize objects, scenes, and features within the images processed. It can involve a variety of techniques depending on the end application, including bounding boxes, segmentation, and tagging.
The Importance of Image Annotation in Machine Learning
The relevance of image annotation cannot be overstated. Machine learning models rely heavily on high-quality, well-annotated data to learn and make predictions. Without proper annotation, models can misinterpret visual data, leading to inaccurate outcomes. Here are a few reasons why image annotation is vital:
- Improves Accuracy: Well-annotated data increases the algorithm's ability to generalize from training data, which enhances its predictive accuracy.
- Facilitates Deep Learning: Deep learning models require massive amounts of labeled data to perform effectively, making image annotation essential.
- Enhances Object Detection: Annotation allows machines to understand where objects are located within images, which is crucial for applications in surveillance, retail, and autonomous vehicles.
- Boosts Efficiency: Automation in image annotation streamlines workflows, saving time and reducing human error.
Types of Image Annotation Techniques
Various image annotation techniques cater to different machine learning tasks. Understanding these techniques is crucial for selecting the right approach for a specific application. Here are the primary types of image annotation:
1. Bounding Box Annotation
This is one of the most common forms of image annotation, where simple rectangular boxes are drawn around objects of interest. It provides clear, concise labeling for tasks such as object detection.
2. Semantic Segmentation
In semantic segmentation, each pixel of an image is classified into predefined categories. This allows for a more detailed understanding of the scene and is particularly useful in applications such as medical imaging and autonomous driving.
3. Instance Segmentation
Similar to semantic segmentation, instance segmentation differentiates between separate objects within the same category (e.g., individual cars in a parking lot). This is useful for tasks requiring identification of unique objects.
4. Keypoint Annotation
Keypoint annotation involves marking specific points on objects (such as a person's joints or facial features) to assist in pose recognition and facial recognition tasks.
5. Polygon Annotation
Polygon annotation is utilized for irregularly shaped objects, allowing for precise labeling that goes beyond simple rectangles, which is indispensable in more complex image data.
Challenges in Image Annotation
While image annotation is essential, it is not without its challenges. Here are a few notable ones:
- Scalability: Annotating large datasets can be a daunting task, requiring significant time and resources.
- Quality Control: Human errors can impact the quality of annotations, necessitating rigorous quality assurance processes.
- Consistency: Maintaining consistent annotation standards across different teams or domains is crucial for effective machine learning outcomes.
- Cost: Outsourcing annotation tasks can be expensive, and computation resources need to be considered for larger data sets.
Choosing the Right Data Annotation Tool
With numerous options available in the market, selecting the right data annotation tool can be challenging. Tools should facilitate efficient workflows, provide quality assurance features, and support various annotation types. One leading provider in this domain is keylabs.ai, offering a comprehensive suite of features:
Key Features of keylabs.ai
- Intuitive Interface: A user-friendly interface that simplifies the annotation process for both technical and non-technical users.
- Collaborative Workflow: Enables teams to work simultaneously on data annotation projects, improving productivity and efficiency.
- AI-Assisted Annotation: Incorporates automated tools that speed up the annotation process while maintaining accuracy.
- Quality Assurance: Includes tools for validating and verifying annotations to ensure high data quality.
- Scalability: Capable of handling large volumes of data annotation projects without compromising performance.
The Future of Image Annotation for Machine Learning
The landscape of image annotation for machine learning is continuously evolving. As AI technologies advance, we can expect several trends to shape the future of this field:
- Increased Automation: AI-driven annotation tools will become more prevalent, allowing for faster and more accurate processing of image data.
- Enhanced Customization: Annotation platforms will offer customizable features to cater to specific project requirements.
- Integration with Other Technologies: Annotation tools will increasingly integrate with other technologies such as cloud computing and edge computing for seamless data handling.
- Sustainability Focus: As businesses prioritize sustainability, annotation processes will evolve to align with eco-friendly practices.
Conclusion
Image annotation is a fundamental component of successful machine learning projects. By employing effective annotation techniques and leveraging platforms like keylabs.ai, businesses can significantly enhance their AI capabilities. As machine learning continues to transform industries, investing in high-quality image annotation is not just advantageous – it is essential for staying competitive in the digital landscape.
In an era where data drives decision-making, ensuring that your image annotation for machine learning strategies are robust and effective can make all the difference. The future is here, and those who embrace the power of effective image annotation will undoubtedly lead the charge in the technological advancements of tomorrow.