Essential Labeling Tools for Machine Learning
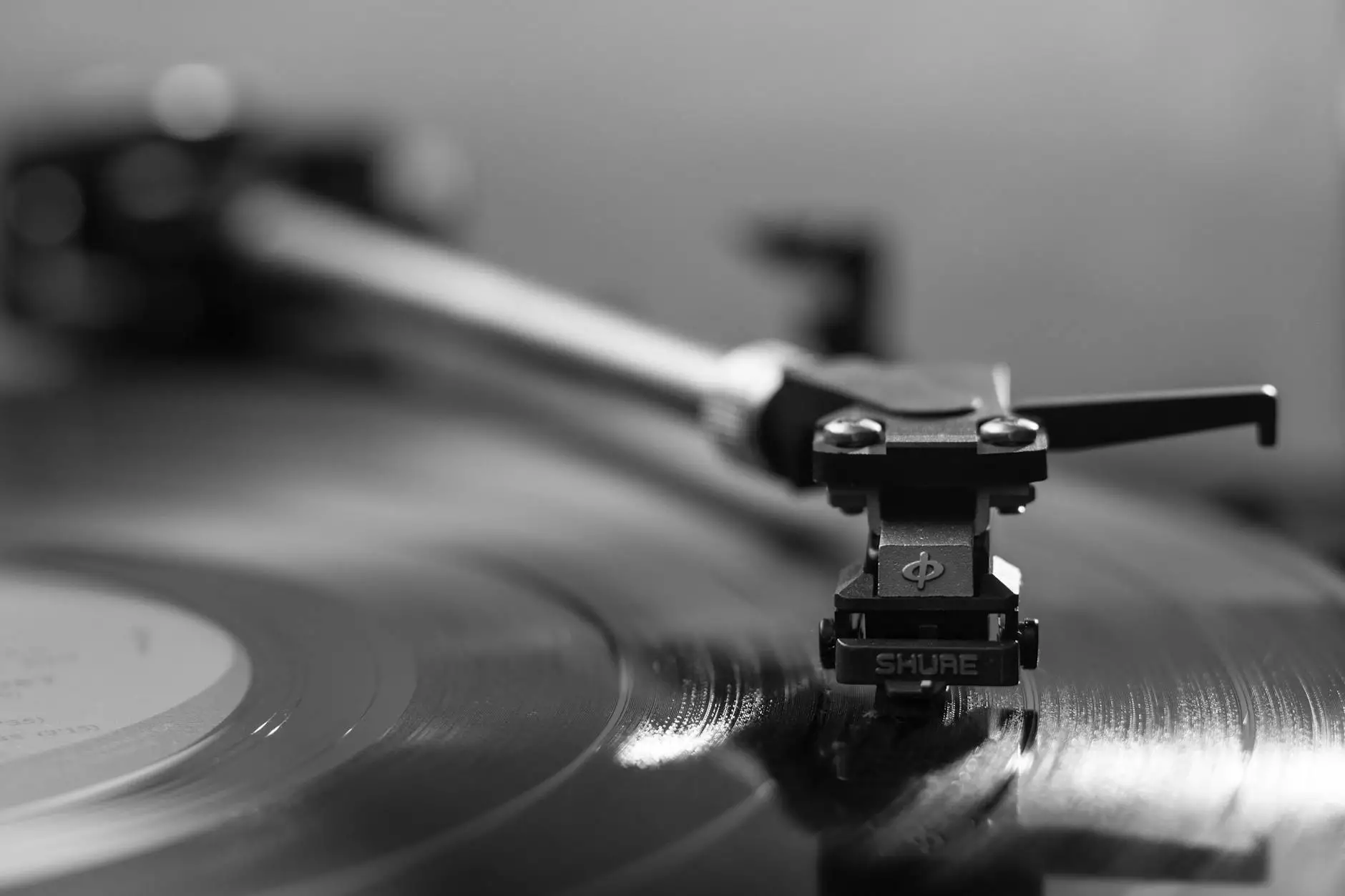
In the fast-evolving world of machine learning, the significance of high-quality data cannot be overstated. As we venture deeper into the realm of artificial intelligence, the need for precise data annotation increases exponentially. This process, commonly referred to as labeling tools for machine learning, is a critical component that directly impacts the performance of machine learning models.
Understanding the Importance of Labeling Tools
Data labeling is the backbone of machine learning projects. It involves tagging data with relevant information, enabling algorithms to learn from various datasets and make predictions or classifications. The accuracy of the labeling directly correlates with the efficiency of the algorithms. Hence, selecting the right labeling tools is vital for your machine learning endeavors.
What Makes a Good Labeling Tool?
Choosing the right labeling tools for machine learning can be a daunting task, but several factors can help simplify your decision:
- User-Friendly Interface: The tool should be easy to navigate and comprehend, allowing annotators to work efficiently.
- Scalability: As your data grows, your labeling tool should scale accordingly without compromising performance.
- Customizability: Different projects may require unique labeling criteria. A flexible tool lets you customize workflows and labeling schemas.
- Collaboration Features: Teams often need to work on projects simultaneously; hence, collaborative features can enhance productivity.
- Integration with ML Frameworks: The ability to seamlessly integrate with your existing machine learning frameworks (like TensorFlow or PyTorch) is imperative for a smooth workflow.
- Quality Control Mechanisms: Ensure that your tool offers ways to validate and verify the accuracy of the data labeled.
Top Labeling Tools for Machine Learning
1. KeyLabs.ai Data Annotation Tool
One of the standout products in the market today is the KeyLabs.ai Data Annotation Tool. This powerful platform is engineered specifically for the high demands of machine learning projects and offers a range of features designed to streamline the data labeling process.
Features of KeyLabs.ai
- Versatile Annotation Options: Whether you are working with images, text, or audio, KeyLabs.ai provides versatile tools that cater to various data types.
- Intelligent Automated Tagging: The platform incorporates AI to assist in the labeling process, significantly reducing the manual workload and potential human error.
- Comprehensive Support: KeyLabs.ai offers dedicated support to assist users throughout their data annotation journey, ensuring that they can manage projects effectively.
2. Amazon SageMaker Ground Truth
Amazon's SageMaker Ground Truth is another robust contender in the realm of labeling tools. This service allows users to build highly accurate training datasets for machine learning.
Highlights of SageMaker Ground Truth
- Automated Labeling: Ground Truth can automatically label data using machine learning models, reducing the time spent on the labeling process.
- Data Privacy and Security: Built on Amazon's secure infrastructure, users can be assured their data is well-protected.
- Cost-Effective Solutions: The pay-as-you-go pricing model makes it accessible for businesses of all sizes.
3. Labelbox
Labelbox is gaining popularity due to its user-centric design and powerful features. It’s tailored for teams looking to scale their data labeling efforts efficiently.
Key Features of Labelbox
- Streamlined Workflow: The platform offers a highly intuitive interface that simplifies the annotation workflow.
- Real-Time Collaboration: Teams can work simultaneously, ensuring alignment and enhancing productivity.
- Integrations: Labelbox integrates with various tools and platforms, allowing seamless data management.
Best Practices for Using Labeling Tools in Machine Learning
Using labeling tools for machine learning effectively requires consideration of a few best practices:
1. Define Clear Guidelines
Before starting the labeling process, it’s crucial to define guidelines for annotators. Clear definitions and standards help maintain consistency, ensuring the quality of the labeled data.
2. Train Your Annotators
Investing time in training your data annotators can drastically improve the accuracy of the labels. Consider holding workshops or utilizing training modules to familiarize them with the labeling tool and project requirements.
3. Monitor Quality Regularly
Implement quality control measures to regularly review the accuracy of labeled data. This may involve having a separate team verify labels or using validation techniques integrated within the labeling tool.
4. Emphasize Iteration
Machine learning projects often require iterations. Use insights gained from model training to refine your labeling guidelines continuously. This iterative approach helps optimize the training dataset.
5. Collaborate Across Teams
Facilitating collaboration between data scientists, annotators, and domain experts can enhance the labeling process. Diverse perspectives contribute to better understanding and quality of the labeled content.
Conclusion
In conclusion, the right labeling tools for machine learning can significantly impact the success and efficiency of your machine learning projects. From enhancing data accuracy to streamlining workflows, investing in effective data annotation tools is paramount. As the landscape of machine learning continues to grow, staying updated with innovative tools like KeyLabs.ai will ensure you remain competitive. Embrace the power of quality data, and you will pave the way for successful machine learning applications.
Get Started with KeyLabs.ai Today!
If you're ready to elevate your machine learning projects with a powerful data annotation platform, visit KeyLabs.ai today and explore how our Data Annotation Tool can transform your data labeling experience.